In the beginning
Let’s be clear about Artificial Intelligence. The end game of this pursuit is a walking, talking, thinking “beings” indistinguishable from the flesh and blood variety. Humans have dreamed about and told stories of creating human-like automatons for literally thousands of years. Early philosophers focused on the mind, wondering if there was some sort of universal symbology that could decipher all types of thought. As civilization advanced, fabrication skills became more advanced, various clockwork mechanisms were built to emulate human movement. Some observers thought that mere human-like appearance imbued them with emotions such as anger, compassion, and joy. After all these generations of discussion and exploration of the mind and what constitutes life and thought, when the first digital programmable computer came to fruition in 1940, it seemed that the tools to replicating the human brain were finally at hand.
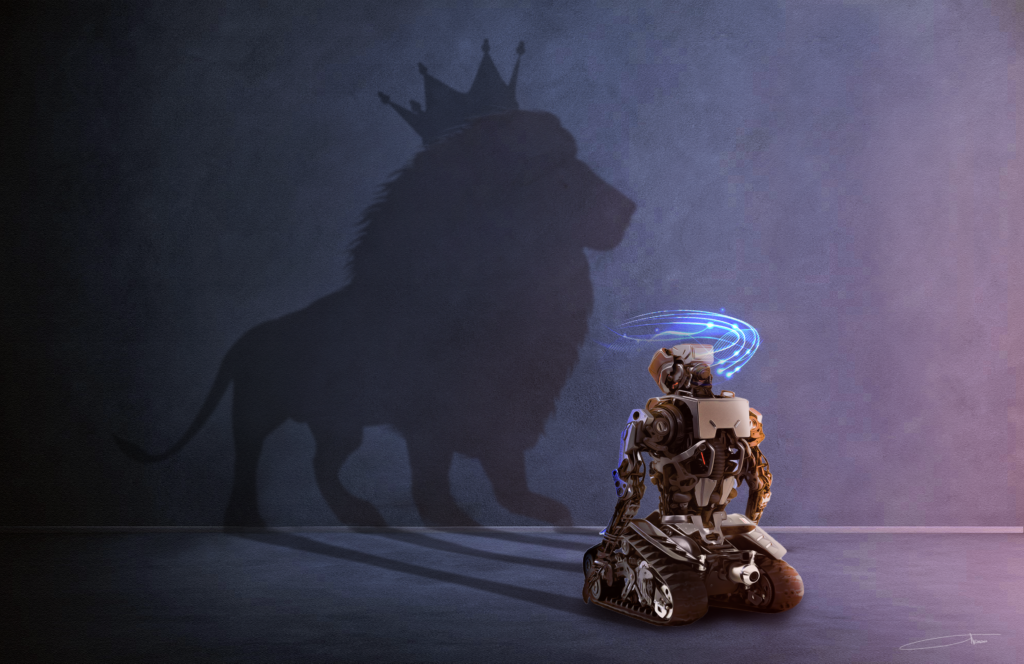
Rise of the Machines
It became clear with this new machine that any formal mathematical process that can be conceived, can be replicated by the mere shuffling of 1’s and 0’s. By extension, any formal thought process could be replicated as well. Academia came together at Dartmouth in the Summer of 1956 specifically to conduct a workshop on this new idea of Artificial Intelligence (as opposed to the natural intelligence of humans and animals). Part of this AI community noted the similarity between the two-state process of digital calculation and the on-off nature of neurons firing in the human brain. Thus, some of the first attempts at AI were focused on building a neural network of on/off switches. What was lacking, though was a working definition that would guide future efforts toward the goal of emulating human thought. Alan Turing proposed the requirement that a human being should be unable to distinguish a machine response from a human being by using the replies to questions put to both. This was sufficiently concise and testable to give the AI community a direction.
The Bubble Bursts
In the years immediately following the Dartmouth meeting, research departments both private and public started producing what was then an astonishing array of advances in hardware and software. It seemed like anything that was conceived could be created. This frenzy of activity and the optimism of the participants attracted lots of venture capital, not the least of which came from DARPA – the US Defense Agency that supports advanced research projects. This was the first AI bubble and people were predicting that within one generation they would have a working computer brain. Four centers of excellence emerged during this time; MIT AI Lab (swallowed up by DARPA), Stanford AI Project, Edinburgh University, and Carnegie Mellon University. Ultimately, these research efforts were stymied by limited computing power which was many orders of magnitude too slow to process simple inputs. This put hard limits on speech recognition, vision systems and ability to “reason” – all elements deemed necessary to pass a Turing test. By the mid 70’s, the lack of progress in machine intelligence meant AI funding was evaporating quickly.
Can Computers Think?
With the bursting bubble, philosophical battle lines were drawn. Perhaps one of the most contentious is whether the objective of true AI was human-like responses to environmental inputs, or actual “common-sense reasoning”, replicating the human thought process. The lead to the concept of “frames” or “scripts” – a set of characteristics associated with our understanding of the world. For example, a chair frame might include, four legs, a flat area for sitting and a back for support. Not a perfect description but good enough as a starting point. It was also during this time that a consortium of researchers from England and France worked together to develop the first codified programming language (called Prolog) that allowed for uniformity in computer programming. These developments (along with improved cost and speed of memory) paved the way for Expert Systems. This approach bypassed philosophical arguments of common-sense reasoning and focused strictly on creating narrowly focused knowledge bases designed to augment decision making. Such narrow systems as chess-playing computers, analysis of blood samples for disease, interpretation of spectral analysis of materials and so forth emerged at this time and a genuine market emerged.
The Race is on?
With increasing demand for expert systems, growth in research of AI was reinvigorated with investments. The success of this approach to building narrowly focused AI engines, prompted one researcher to propose solving the common-sense problem by building an expert system consisting of all the commonsense knowledge possessed by the average human and accessed through an inference engine. This job of capturing commonsense knowledge has been going on for over three decades and continues today. This approach has found commercial use in language processing, financial services, and healthcare areas. In 1981 the Japanese government, which had their own AI program, announced an $850M investment in their fifth generation AI robot whose goal was to “converse and reason in a natural way”. This triggered a sort of “race to the moon” mentality and governments around the world suddenly found the money to invest in their own robotic programs. Sadly, again the producers had over-promised and under-delivered. Expert programs were expensive to maintain, not easily updated and prone to problems when confronted with unusual inputs. In addition the cost of specialized AI computers was too expensive when compared to the new crop of desktop computers from IBM and Apple. This time the bubble lasted until 1993.
Does AI even exist?
Retrenching in the AI world meant taking the useful learning developed over the last half century and deploying it to a more specific class of problems, by applying rigorous logical and mathematical principles. What has emerged so far is the application of AI principles to specific types of objectives such as deep learning, big data, and predictive analytics. At the same time what was once known as AI is being re-branded into AGI (Artificial General Intelligence – the ability to solve any general problem; plan dinner, open a door, walk the dog). There is a contingent in the AGI community that postulates that the gateway to true or “strong” AI requires robotic development. This creates the ability to “sample” the real world continuously and (it is believed) is the only path to learning how to behave like a human.
So where are we now?
Given the boom-and-bust history of AI, there is reason to be skeptical about the use of the term artificial intelligence. In reality, a clear definition still does not exist for the term. The current working definition in this field is “intelligent agents”, referring to any system that perceives its environment and takes actions that maximize its chance of achieving its goals. There is no longer widespread support for the Turing definition. And most of the capability offered up as AI is probably more rightly classified as sophisticated computer algorithms rather than embedded intelligence. Researchers still work toward a definition of AGI or intelligent agents. In the meantime, they have produced useful work in fields associated with machine “intelligence”: data mining, speech recognition, search engines, machine learning and logistics are some examples.
Identifying and predicting when the next breakthrough will occur is an impossible task. But perhaps scientists have been looking in the wrong place. The secret to success may ultimately lie in solving for Moravec’s Paradox. Hans Moravec was one of the pioneering thought leaders in the AI community from the Dartmouth days. He observed that, contrary to traditional assumptions, reasoning requires very little computation, but sensorimotor and perception skills require enormous computational resources. With this observation, Moravec relegates the brain to a repository for all of the highly processed sensory inputs of the body. Perhaps the real intelligence is all of the body’s external stimulation, once it has been filtered through the “common sense engine” that is our brain. Whatever it turns out to be, you can bet companies will continue to tout their latest AI-powered development, at least until the next thing comes along.
Very nice article,Human brain contain 20 Million cells,,There are not enough scientists in the world,that has the ability to research it,not even The axons of the brain,but only relatively few,at least We understand the functions of the brain’s cells axons’ & dendrites,as well as Brain’s Biochemicals actions.and reaction(Biochemical messengers) etc.